As we discussed in our previous methods note introducing the concept of heterogeneous treatment effects (HTEs), understanding whether the effects of an exposure or treatment are different for different people is essential to population health research, especially health equity research. Here we discuss some of the research challenges in evaluating HTEs and priorities for future research about HTEs.
by Ellicott C. Matthay, PhD
Published July 24, 2020
What do we know about HTEs?
Evaluation of HTEs is often omitted entirely or considered largely as an afterthought in health research. Exploratory research on HTEs is often unreported and therefore unconfirmed. As a result, existing evidence on the prevalence of HTEs is noisy at best and nonexistent or unreliable at worst. There is substantial uncertainty about how frequently large differences in treatment effects occur across population subgroups and what characteristics define those subgroups (e.g., age, socioeconomic status).
For a variety of topics, theory would suggest that HTEs are likely and empirical research exists to support those theories. For example, theories of resource substitution suggest that people who are deprived of multiple health-promoting resources—such as education, income, and power—will reap greater benefits from access to any one of these resources than individuals who face fewer barriers.1 Supporting this, Vable and colleagues found that those with low childhood socioeconomic status benefitted more from each additional year of education. Other theories that may support HTEs include intersectionality, structural barriers, and complementarity of resources.2,3
HTEs seem especially plausible for many social exposures because of the complex, multi-level, and dynamic interrelations among social factors.4 Indeed, HTEs have been documented for a variety of social exposures that are of particular interest to E4A. HTEs are also of considerable interest in clinical care, as evidenced by growing emphasis in personalized or precision medicine. Yet systematic approaches to assessing HTEs remain a challenge.
Challenges in evaluating HTEs
Estimates of treatment effects in population subgroups are intrinsically less precise than estimates for the whole population, so chance findings are more likely. Reports of HTEs—even within the context of randomized trials—are therefore often viewed skeptically. Unless specific factors were pre-specified as likely to define groups with differential treatment effects, evidence of HTEs may be considered subject to cherry picking.
This issue is likely to receive more attention as machine learning algorithms that identify complex interactions—including those related to HTEs—become more common. Machine learning tools may accelerate discovery of novel determinants of health and enhance theoretical understanding of the drivers of health inequalities, but to date these tools have been adopted in few applied studies of social factors and health.5 The slow uptake may partly be due to controversy about how to balance the threat of fishing and false discovery with the goal of acknowledging uncertainty and pursuing true exploration of novel risk factors. Further, there is a long-standing emphasis on a priori hypothesis specification before testing for HTEs, which flies in the face of machine learning methods.
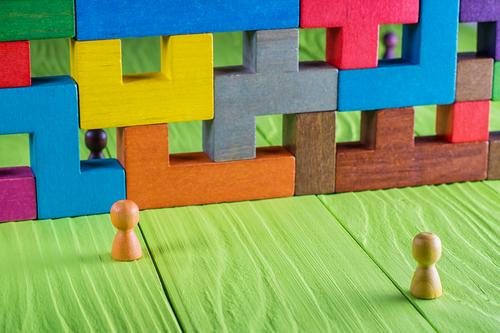
It is impossible to assess whether a treatment effect differs for a particular type of person if there is no such person in the study sample.
Fully evaluating HTEs is challenging in study samples that are less diverse than the population: it is impossible to assess whether a treatment effect differs for a particular type of person if there is no such person in the study sample. This is why study samples that reflect the full diversity of the population are desirable, and especially important when evaluating inequalities. With heterogeneous participants, we can fully evaluate differential effects across population subgroups. Understanding these differential effects is important for a number of reasons (see Part 1 of this Methods Notes series).
Another major challenge is that the degree of heterogeneity depends on the scale on which effects are defined—additive or multiplicative. By additive scale, we mean absolute estimates that describe how many extra cases would occur if the population were exposed versus not exposed. Typical difference measures such as the risk difference or rate difference fall in this category. By multiplicative scale, we mean the relative or percent change in number of cases if the population were exposed versus not exposed. Ratio measures such as the odds ratio and relative risk fall in this category. If a treatment reduces the probability of an adverse health outcome, there may be a large number of cases prevented if the condition is common or a small number if the condition is rare. When we evaluate whether and how much the effect of treatment differs based on a 3rd characteristic (e.g., race), the answer will depend on whether we characterize the effect on an additive or multiplicative scale.
If both the exposure and the hypothesized modifier have independent effects on the outcome, and there is no heterogeneity in effects on the additive scale—for example in the attributable risk or risk difference—then by definition, there will be heterogeneity in effects on the multiplicative scale—for example in the relative risk.6 In epidemiology, which scale is more appropriate for assessing HTEs and consequently, whether HTEs even exist, continues to be a source of major controversy. For issues of resource priorities, however, the additive scale is most relevant.
Open questions
At E4A, we are interested in determining what works best, for whom, and under what circumstances; HTEs are at the heart these questions. Yet we face tradeoffs in pursuing HTE-related research questions given data limitations. Given limited resources, E4A studies often rely on existing secondary data, some of which isn’t collected or disaggregated by subgroup. Sometimes samples are too small to detect meaningful changes for subgroups. When large samples are required to detect HTEs with adequate power, how do we weigh the extra expense versus the knowledge gained? Should we have a strong preference for studies that incorporate sampling strategies and sufficient power to allow for formal evaluation of effect heterogeneity or is including diverse study samples sufficient?
We believe there is important work to be done on a range of research questions related to HTEs. Best practices have yet to be identified. Research to develop consensus on what HTEs to evaluate, and how to evaluate, report, and use evidence on HTEs is a priority. Questions include:
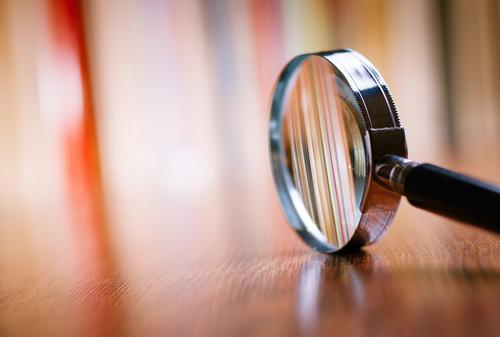
What HTEs to evaluate
When and for whom should HTEs be assessed? For all studies? For all possible subgroups? To establish best practices for this, we need to understand:
- How often does treatment effect heterogeneity happen? For what types of social interventions? Along what dimensions or for what subgroups?
- How often is the heterogeneity trivial? How often is it substantial enough to alter recommendations for policy or practice? If effects differ somewhat but are at least the same sign for everyone in the population, it may not be as important to precisely quantify heterogeneity. But if an intervention may harm some people while helping others, it is essential to understand this.
How to evaluate HTEs
What methods should be used for evaluating HTEs? There is a conceptual separation between methods based on specifying hypothesized subgroups a priori and methods that identify subgroups using data-driven algorithms. Which of these are the most rigorous and appropriate for studying HTEs of social programs and policies? Should all evaluations of HTEs be pre-registered? Which methods are most robust when sample sizes are limited, as they often are for research on social programs and policies?
How to report HTEs
What reporting guidelines should exist for studies assessing HTEs? Should null results be routinely reported for all a priori specified groups and for exploratory analyses that were not pre-specified?
How to use HTE evidence
How should evidence of treatment effect heterogeneity be used when making decisions about policy or practice? How should decisionmakers weigh evidence of unequal benefits or harms?
While research to answer most of these questions falls outside the bounds of what E4A funds, answers to these questions will inform the research designs of E4A applicants, guide E4A’s decision-making regarding HTE-related proposals, and strengthen the methodological foundations of population health research.
References
- Ross CE, Mirowsky J. Sex differences in the effect of education on depression: Resource multiplication or resource substitution? Soc Sci Med. 2006;63(5):1400-1413. doi:10.1016/j. socscimed.2006.03.013.
- Bauer GR. Incorporating intersectionality theory into population health research methodology: Challenges and the potential to advance health equity. Soc Sci Med. 2014;110:10-17. doi:10.1016/j.socscimed.2014.03.022.
- Krieger N. Theories for social epidemiology in the 21st century: An ecosocial perspective. Int J Epidemiol. 2001;30(4):668-677. doi:10.1093/ ije/30.4.668.
- Galea S, Riddle M, Kaplan GA. Causal thinking and complex system approaches in epidemiology. Int J Epidemiol. 2010;39(1):97-106. doi:10.1093/ije/dyp296.
- Glymour MM, Nguyen Q, Matsouaka R, Tchetgen Tchetgen EJ, Schmidt NM, Osypuk TL. Does mother know best? Treatment adherence as a function of anticipated treatment benefit. Epidemiology. 2016;27(2):265-275. doi:10.1097/ EDE.0000000000000431.
- Greenland S, Lash TL, Rothman KJ. Chapter 5: Concepts of Interaction. In: Modern Epidemiology. Third. Lippincott Williams & Wilkins; 2008.

The E4A Methods Lab was developed to address common methods questions or challenges in Culture of Health research. Our goals are to strengthen the research of E4A grantees and the larger community of population health researchers, to help prospective grantees recognize compelling research opportunities, and to stimulate cross-disciplinary conversation and appreciation across the community of population health researchers.
Do you have suggestions for new topics for briefs or training areas? Email us at evidenceforaction@ucsf.edu.