Nearly all research questions of interest to Evidence for Action (E4A) relate to establishing causality: if we intervene to change some system, policy, or action, will this deliver improvements in health or health equity? Quantitative methods for evaluating causal research questions, as opposed to questions that are merely predictive or descriptive, have developed rapidly since the 1980s. There are now myriad methods, each with various advantages and disadvantages. Despite the seemingly immense number of options, most study designs fall into one of two categories, which we label instrument-based or confounder-control. The assumptions required to estimate causal effects for each approach are distinct.
by Ellicott C. Matthay, PhD
Published November 19, 2019
Confounder-Control Studies
In confounder-control studies1, researchers compare outcomes for people observed to have differing treatments and use statistical adjustment to account for differences in characteristics between treatment groups (e.g., age, education, income, etc.). In modern frameworks, bias due to these differences is commonly known as confounding and arises from shared causes of the treatment and the outcome (Figure 1). If left uncontrolled, confounding factors distort the measured association between the treatment on the outcome so the association cannot tell us about the causal effect of treatment on the outcome. For example, if uncontrolled, differing levels of educational attainment could confound the observed impact an employment intervention on mortality. Controlling for confounders allows the researcher to isolate the causal effect of interest (from the treatment to the outcome). The vast majority of observational health studies use some version of confounder-control, such as multiple regression, propensity score matching, or inverse probability weighting. These methods rely on the assumption that confounding factors have been identified, measured, and appropriately controlled. Confounder-control methods can deliver valid causal effect estimates when this assumption is met. These methods are useful when meeting this assumption seems feasible or when a study can improve on the identification, measurement, or control of confounders compared to previous studies.
Figure 1
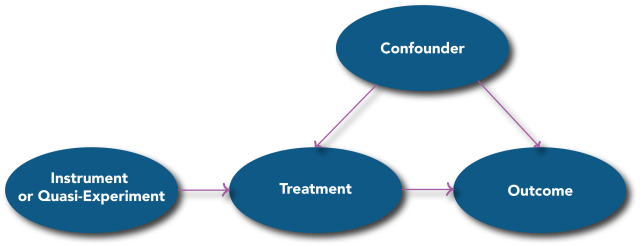
- Rothman KJ, Greenland S, Lash TL. Modern Epidemiology. Philadelphia, PA: Lippincott, Williams & Wilkins 2008.
Instrument-Based Studies
In contrast, in instrument-based studies2, researchers leverage an external or “exogenous” source of variation—often a change in a program, policy, or other accident of time and space—that influences treatment received but is not likely to be otherwise associated with the outcome or other confounders that could be related to the outcome. Many instrument-based study designs can be described as “quasi-experimental”, although this term has been used inconsistently in prior research. Sources of instruments include wait lists (sometimes used to assign resources when there is not enough for all eligible individuals), arbitrary variation in the timing or eligibility criteria of new programs or policies, or geographic proximity to facilities or services (which affects the use or uptake of the services).
For example, arbitrary variation in the introduction of compulsory schooling laws (CSL) across states and time affected the number of years of schooling residents received. In order to evaluate the effect of years of schooling on mortality, researchers have argued that CSL introduction is an “exogenous variable” that influences years of schooling, but is otherwise unrelated to mortality or shared causes of mortality and schooling (confounders) (Figure 1)3–5. Thus, differences in CSL introduction can be used to study differences in years of schooling, and in turn evaluate the effects of schooling on mortality, that are unrelated to and unbiased by confounders such as characteristics of the individual. If CSLs increase education but do not lead to differences in mortality, it implies that schooling does not affect mortality. If differences in CSLs predict large differences in mortality, it implies that schooling has large effects on mortality. Various statistical formulas use the magnitude of the association between CSL introduction and mortality to estimate the magnitude of the effect of schooling on mortality.
When studying health outcomes, the most commonly applied instrument-based approaches include regression discontinuity, instrumental variables, or differences-in-differences methods. All of these methods rely on two assumptions, intuitively: (1) there must be an exogenous factor which influences the treatment, and (2) this factor must have no other reason to be associated with the outcome except via its effect on the treatment. The major challenge in fielding an instrument-based study is identifying such an exogenous factor. In some situations, the exogenous variable only meets the assumptions within a small segment of the population or after accounting for other factors, such as long-term temporal trends. These types of caveats may be addressable with statistical adjustment. For example, residential proximity to a clinic may affect service uptake, but where people live also determines other exposures affecting health that must be controlled. Additionally, instrument-based approaches usually have less statistical power than confounder-control analysis of the same data and thus require larger sample sizes. These methods also only tell us about effects of exposure in the subset of people whose treatment was affected by the exogenous factor—for example, those whose treatment was strictly due to the policy change or who were right at the cusp of eligibility thresholds.
- Angrist JD, Pischke J-S. Mostly Harmless Econometrics: An Empiricist’s Companion. Princeton University Press 2008.
- Lleras-Muney A. Were Compulsory Attendance and Child Labor Laws Effective? An Analysis from 1915 to 1939. The Journal of Law and Economics 2002;45:401–35. doi:10.1086/340393
- Lleras-Muney A. The Relationship Between Education and Adult Mortality in the United States. The Review of Economic Studies 2005;72:189–221. doi:10.1111/0034-6527.00329
- Hamad R, Elser H, Tran DC, et al. How and why studies disagree about the effects of education on health: A systematic review and meta-analysis of studies of compulsory schooling laws. Social Science & Medicine 2018;212:168–78. doi:10.1016/j.socscimed.2018.07.016
E4A Funded Studies
As of this publication, E4A has funded many instrument-based or similarly designed studies. For example, one project is using the opening of community colleges in a particular place as a quasi-experiment to evaluate the effects of attending community college; another uses a lottery that provided public housing vouchers to eligible families to evaluate the health effects of tenant-based versus project-based housing assistance; a third evaluates health outcomes for low-income families in homes receiving weatherization treatments, comparing families who received the intervention first versus those who were wait-listed for later housing improvements. However, identifying that there is an opportunity to evaluate a program or policy using instruments or similar methods is also challenging and requires a detailed understanding of how and why people come to be treated (or exposed) in a given community. For example: is assignment to a waitlist (and subsequent receipt of resources), truly random, or is there some type of prioritization or personal bias that influences position on the list? Did anything besides community college access change at the same time new colleges were opened? For this reason, we actively encourage practitioners with detailed knowledge of a program or policy to submit an application if they see a possible instrument-based happening in their own community that could be leveraged for research.
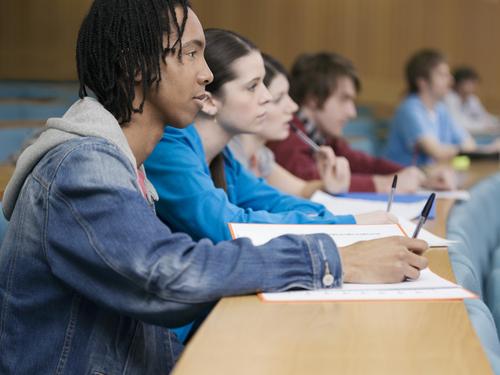

The E4A Methods Lab was developed to address common methods questions or challenges in Culture of Health research. Our goals are to strengthen the research of E4A grantees and the larger community of population health researchers, to help prospective grantees recognize compelling research opportunities, and to stimulate cross-disciplinary conversation and appreciation across the community of population health researchers.
Do you have an idea for a future methods note? Contact us and let us know.